The neonatal unit is a busy environment where every second matters and every decision counts. The challenge is how do we support the demands of research in this environment when the daily demands are daunting. Perhaps Ai can help?
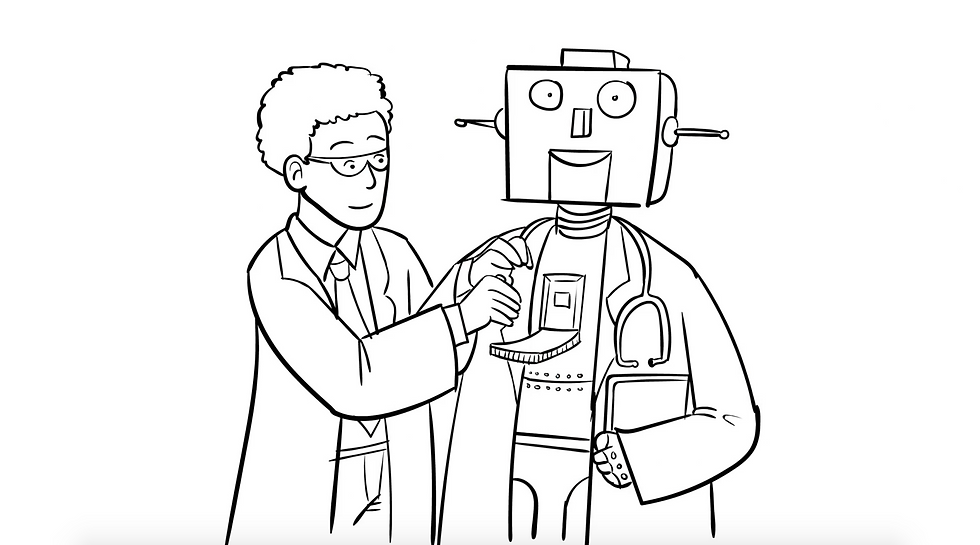
Necrotizing enterocolitis (NEC) is a severe disease that affects the gastrointestinal (GI) tract of premature infants. Different areas of NEC research have often been isolated from one another and progress on the role of the inflammatory response in NEC, on the dynamics of epithelial layer healing, and on the positive effects of breast feeding have not been synthesized to produce a more integrated understanding of the pathogenesis of NEC. We seek to synthesize these areas of research by creating a mathematical model that incorporates the current knowledge on these aspects.
The power of newer data processing systems
Modelling enabled by machine learning can fully capture the complexities created by several aetiologies that interact and overlap. Most importantly it can still retain the capacity to explain, in a straightforward manner, how particular diagnostic insight has been reached to the reviewing consultant.
Unlike previous models that are based on ordinary differential equations, our mathematical model takes into account not only transient effects but also spatial effects. A system of nonlinear transient partial differential equations is solved numerically using cell-centered finite differences and an explicit Euler method. The model is used to track the evolution of a prescribed initial injured area in the intestinal wall. It is able to produce pathophysiologically realistic results; decreasing the initial severity of the injury in the system and introducing breast feeding to the system both lead to healthier overall simulations, and only a small fraction of epithelial injuries lead to full-blown NEC. In addition, in the model, changing the initial shape of the injured area can significantly alter the overall outcome of a simulation. This finding suggests that taking into account spatial effects may be important in assessing the outcome for a given NEC patient. This model can provide a platform with which to test competing hypotheses regarding pathological mechanisms of inflammation in NEC, suggest experimental approaches by which to clarify pathogenic drivers of NEC, and may be used to derive potential intervention strategies.
The power of ensemble modelling
The data should be applied to Artificial Intelligence modelling, as deep learning has the capability to advance our understanding beyond the limitations of looking at a single variable but can objectively capture the complexities of real-world scenario. Deep learning simultaneously enables multiple algorithms in pathophysiologic reasoning, disease incubation period simulation, image pattern recognition and models all variables to the specific patient's presentation to narrow the number of diagnostic possibilities.
Untapped potential
Application in day to day application in the patient care and unit monitoring promising more factual decision making, ultimately making timelier interventions to improve patient care outcomes
Such modelling can fully capture the complexities created by several aetiologies that interact and overlap. Most importantly it can still retain the capacity to explain, in a straightforward manner, how particular diagnostic insight has been reached to the reviewing consultant. Ongoing feedback loop (natural degradation and optimisation of neural networks) shows great promise to improve and simplify current diagnostic criteria’s. This latter ability shows a further unexplored application in day to day application in the patient care and unit monitoring promising more factual decision making, ultimately making timelier interventions to improve patient care outcomes.
Comments